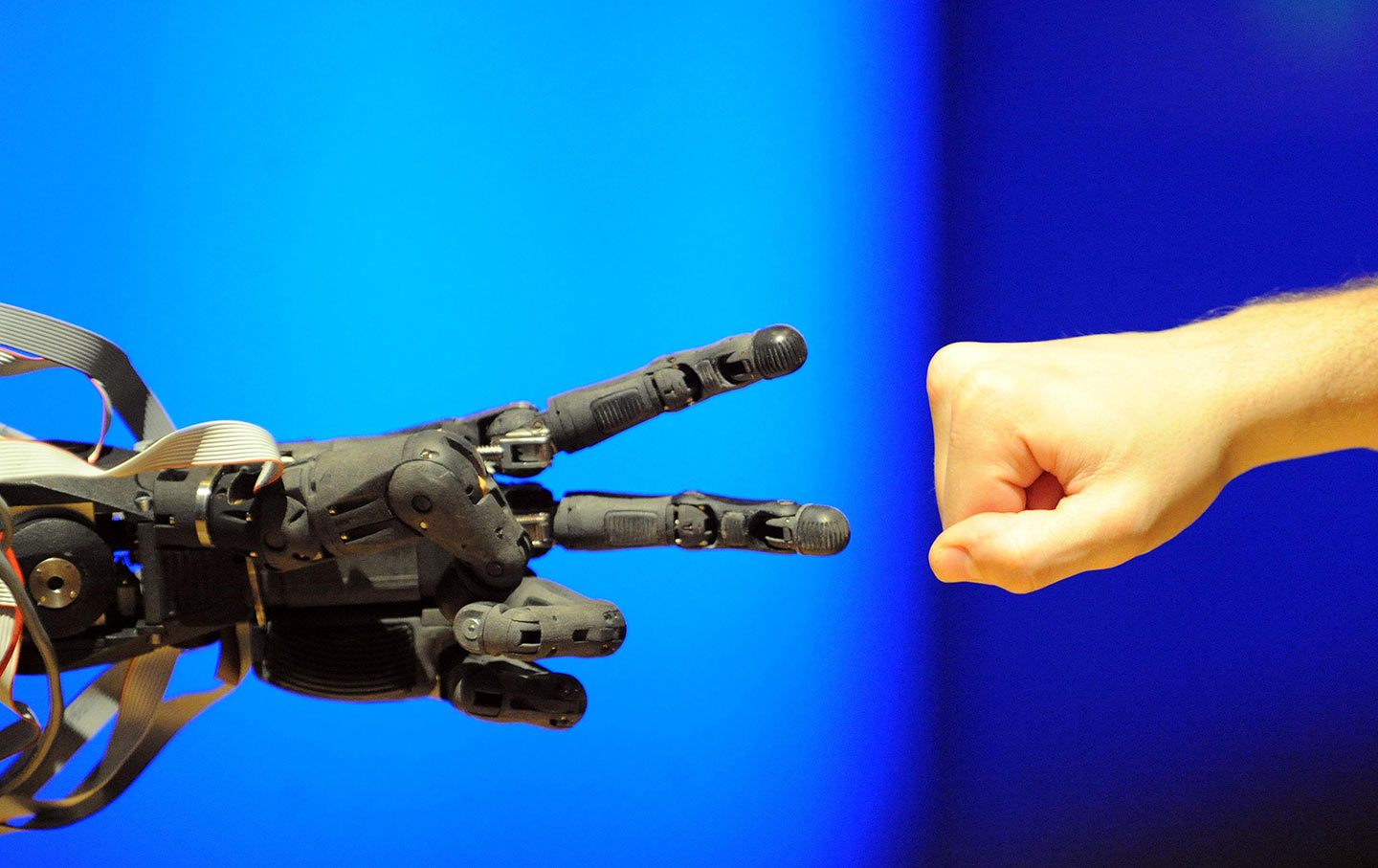
"One of my favorite things about the tech industry is how quickly innovations from the big companies and premium products trickle down into more affordable devices. The rampant stealing of ideas isn't so awesome when it happens between small companies — or, as in the case of Facebook treating Snapchat like its incubation lab, when a big company copies a smaller one. But I don’t have a problem with the general flow of good ideas from giants like Apple and Google to more budget-friendly suppliers of hardware and software. Apple and Google, though, have an obvious problem with that, and they’ve worked hard to develop new techniques and approaches that can’t be readily imitated.
The big new thing in smartphones lately is one of those buzz phrases you’ll have heard tossed around: machine learning (ML). Like augmented and virtual reality, machine learning is often thought of as a distant promise, however in 2017 it has materialized in major ways. ML is at the heart of what makes this year’s iPhone X from Apple and Pixel 2 / XL from Google unique. It is the driver of differentiation both today and tomorrow; and the companies that fall behind in it will find themselves desperately out of contention.
A machine-learning advantage can’t be easily replicated, cloned, or reverse-engineered: to compete with the likes of Apple and Google at this game, you need to have as much computing power and user data as they do (which you probably lack) and as much time as they’ve invested (which you probably don’t have). In simple terms, ML promises to be the holy grail for giant tech companies that want to scale peaks that smaller rivals can’t reach. It capitalizes on vast resources and user bases, and it keeps getting better with time, so competitors have to keep moving just to stay within reach.
I’m not arguing that ML is a panacea any more than I would argue that all OLED displays are awesome (some are terrible): it’s just the basis on which some of the key differentiating features are now being built.
Google’s HDR+ camera
Let’s start with the most impressive expression of machine-learning consumer tech to date: the camera on Google’s Pixel and Pixel 2 phones. Its DSLR-like performance never ceases to amaze me, especially in low-light conditions. Google’s imaging software has transcended the traditional physical limitations of mobile cameras (namely: shortage of physical space for large sensors and lenses), and it’s done so through a combination of clever algorithms and machine learning. As Google likes to put it, the company has turned a light problem into a data problem, and few companies are as adept at processing data as Google.
I spoke with Marc Levoy, the Stanford academic that leads Google’s computational photography team, recently, and he stressed something important about Google’s ML-assisted camera: it keeps getting better over time. Even if Google had done nothing whatsoever to improve the Pixel camera in the time between the Pixel and Pixel 2’s launch, the simple accumulation of machine learning time will have made the camera better. Time is the added dimension that makes machine learning even more exciting. The more resources you can throw at your ML setup, says Levoy, the better its output becomes, and time and processing power (both on the device itself and in Google’s vast server farms) are crucial.
Related
Google’s Assistant
At CES in January this year, Huawei’s mobile boss Richard Yu was asked if his company would introduce its own voice assistant in the US, to which he replied, “Alexa and Google Assistant are better, how can we compete?” That uncharacteristically pragmatic response (for a mobile company CEO) neatly encapsulates the difficulty of copying Google and Amazon’s machine-learning efforts. All the vast resources that the two US companies have invested into natural language processing and voice recognition are returning a dividend in keeping them far enough ahead of the competition that even Huawei, one of the biggest consumer tech brands outside the US, isn’t trying to compete. That’s the cumulative power of long-term investment in machine learning.
Is Google Assistant a differentiating feature? Not for hardware, as Google wants to have Assistant running on every device possible. But the Assistant serves as a conduit for funneling users into Google search and the rest of the company’s services, with practically all of them benefiting from some variety of machine learning, whether you’re thinking of Google Maps tips or YouTube video suggestions. What Assistant does for the mobile market is to enhance Google’s influence over its hardware partners: woe betide the manufacturer that tries to ship an Android phone in 2018 without either the Google Play Store or Assistant on board.
Apple’s Face ID
On the Apple side of the fence, machine learning is permeating much of the software running on the iPhone already, and the company’s Core ML tools are making it easy for developers to add to that library. But the big highlight feature of the new iPhone X, the thing everyone notices, is the notch at the top of its display and the technology contained within it. Up in that monobrow section, you’ll find a full array of infrared and light sensors, something tantamount to a Microsoft Kinect system, which facilitates the new FaceID authentication method.
I remain uncertain about how well Face ID strikes the balance between security and convenience (especially without the fallback of Touch ID’s fingerprint recognition), but I have no doubt about the technical achievement that it represents. Everyone I know that has used Face ID gives a glowing assessment of its accuracy. The system is robust enough to work in the dark and, thanks to machine learning, it will adapt to changes in your appearance. If you strip away all the usual incremental upgrades and design tweaks, the FaceI D system is the iPhone X’s defining new feature. And it’s reliant on ML to work its technological magic..."
Democracy Needs a Reboot for the Age of Artificial Intelligence | The Nation